A sort of artificial intelligence technology known as “deepfake” creates synthetic material, including audio, video, and photos, using machine learning techniques, especially generative adversarial networks (GANs). Creating extremely lifelike synthetic media that mimic real people while manipulating some features of the content is the aim of deepfake technology. Deep learning and generative adversarial networks are the two methods that underpin deepfake technology. Artificial neural networks, a type of algorithm that is inspired by the structure and operation of the brain, are used in the machine learning discipline of deep learning to process and analyze massive amounts of data. Numerous fields, including robotics, computer vision, natural language processing, and speech recognition, have benefited from the use of deep learning.
Generative adversarial networks (GANs) are a machine-learning approach used to construct deepfakes. A generator and a discriminator neural network, which make up a GAN, are trained using a sizable dataset of real photos, videos, or audio. The generator network generates artificial data that mimics the actual data in the training set, like a synthetic image. After that, the discriminator network evaluates the veracity of the synthetic data and gives the generator input on how to enhance its output. Until the generator generates synthetic data that is remarkably lifelike and challenging to discern from the real data, this process is repeated numerous times, during which the discriminator and generator learn from one another. Using this training set, deepfakes are produced. which may be applied in various ways for video and image deep fakes:
(a) face swap: transfer the face of one person for that of the person in the video;
It is crucial to remember that deepfake technology is always developing, meaning that to stay current with the most recent advancements, deepfake detection algorithms must be updated regularly. At the moment, the most effective method for figuring out whether a piece of media is a deepfake is to combine many detection methods, and you should be wary of anything that looks too good to be real. The following are a few of the most popular methods for spotting deep fakes:
- Visual artefacts: Some fakes have noticeable visual artefacts, such as unnatural facial movements or blinking, that can be a giveaway that the content is fake. Visual artefacts in deepfakes can arise due to several factors, such as limitations in the training data, limitations in the deep learning algorithms, or the need to compromise between realism and computational efficiency. Some common examples of visual artefacts in deepfakes include unnatural facial movements or expressions, unnatural or inconsistent eye blinking, and mismatched or missing details in the background.
- audio-visual mismatches: In some deepfakes, the audio and visual content may not match perfectly, which can indicate that the content has been manipulated. For example, the lip movements of a person in a deepfake video may not match the audio perfectly, or the audio may contain background noise or echoes that are not present in the video. These types of audio-visual mismatches can be a sign that the content has been manipulated.
- Deep learning-based detection: Deep learning algorithms, such as deep neural networks, can be used to detect deepfakes by training the algorithms on a large dataset of real and fake images, videos, or audio. The algorithm learns the patterns and artefacts that are typical of fake content, such as unnatural facial movements, inconsistent eye blinking, and audio-visual mismatches. Once the deep learning algorithm has been trained, it can be used to detect deepfakes by analysing new, unseen media. If the algorithm detects that a piece of media is fake, it can flag it for manual inspection or flag it for further analysis.
- Forensics-based detection: Forensics-based detection methods involve analysing various aspects of an image or video, such as the geometric relationships between facial features, to determine whether the content has been manipulated or not. These methods can be effective in detecting deepfakes, but they are not foolproof. Deepfake creators are constantly improving their techniques to make the forgeries more convincing, so forensic methods need to be constantly updated to keep up. In addition to analysing facial features, other forensic methods include analysing patterns in audio and video data and examining inconsistencies in lighting, shading, and other visual cues. These methods can help identify deepfakes, but they are not a guarantee, and there is always a possibility that a sophisticated deepfake may go undetected.
- Multi-model ensemble: The use of a multi-model ensemble is a popular approach in deepfake detection as it can provide more accurate results compared to using a single model. By combining the outputs of multiple deepfake detection methods, a multi-model ensemble can provide a more comprehensive evaluation of the authenticity of an image or video. For example, one model might focus on analysing the geometric properties of facial features, while another might focus on detecting inconsistencies in lighting and shading. By combining the results from these models, a multi-model ensemble can provide a more robust evaluation of the authenticity of the content.
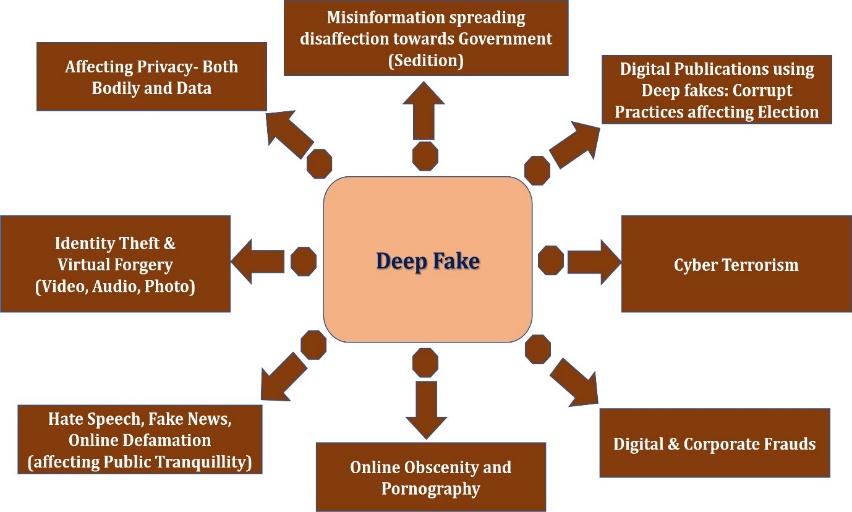
Illustrations of various crimes committed using deepfakes
The following crimes can be committed using deepfake:
- Identity theft and virtual forgery: Identity theft and virtual forgery using deepfakes can be serious offences and have significant consequences for individuals and society as a whole. Deepfakes can damage a person’s reputation and credibility and disseminate misleading information if they are used to steal someone’s identity, incorrectly portray them, or sway public opinion. These offences are punishable under Section 66-C (penalty for identity theft) and Section 668 (computer-related offences) of the Information Technology Act, 2000. In this context, the Penal Code, 1860, Sections 420 and 468, would also be cited.
- Misinformation against governments: It is a severe problem that can have far-reaching effects on society when deepfakes are used to propagate false information, undermine the government, or foster hatred and disenchantment against it. The dissemination of incorrect or misleading information has the potential to sway public opinion, erode public confidence, and affect political outcomes. Cyberterrorism offences fall under the purview of Section 66-F of the Information Technology Act of 2000, as well as the Information Technology (Intermediary Guidelines and Digital Media Ethics Code) Amendment Rules, 2022. In this context, Section 121 of the Penal Code, 1860 (waging war against the Government of India) and Section 124-A may also be used.
- Hate speech and online defamation: Hate speech and online defamation using deepfakes can be serious issues that can harm individuals and society as a whole. In addition to seriously harming people’s reputations and well-being, the use of deepfakes to disseminate hate speech or libellous content can also contribute to a toxic online environment. The Information Technology Act of 2000 permits the prosecution of certain offences under the Information Technology (Intermediary Guidelines and Digital Media Ethics Code) Amendment Rules, 2022. Furthermore, in this context, the Penal Code’s Sections 153-A and 153-B (speech influencing public transit) and Section 499 (defamation) may be used.
- Practices affect elections: The use of deepfakes in elections can have significant consequences and undermine the integrity of the democratic process. Deepfakes have the potential to sway public opinion, impact election results, and disseminate inaccurate or misleading information about political candidates. A lot of nations and organisations are acting to address the growing worry over the influence of fake news on elections. Section 66-D of the Information Technology Act of 2000, which deals with the punishment for cheating by personation utilising computer resources, and Section 66-F, which deals with cyberterrorism, allow for the prosecution of these offences. A “Voluntary Code of Ethics for the General Election, 2019 that could be invoked to tackle the menace” was also released today by the Social Media Platforms and Internet and Mobile Association of India (IAMAI) in conjunction with Sections 123(3-A), 123, and 125 of the Representation of the People Act, 1951, affecting elections in India.
- Violation of privacy, obscenity, and pornography: This technology can be used to create fake images or videos that depict people doing or saying things that never actually happened, potentially damaging the reputation of individuals or spreading false information. Furthermore, deepfakes could be employed for evil intent, including politically motivated disinformation campaigns, non-consensual pornography, or propaganda. When deepfakes are utilised to disseminate misleading information or sway public opinion, they can have detrimental effects on both society as a whole and the individuals whose photos or likenesses are exploited without their permission. The Information Technology Act of 2000 has several sections that can be used to prosecute individuals who commit these crimes. These include Section 66-E, which deals with privacy violations; Section 67, which deals with publishing or transmitting obscene material; Section 67-A, which deals with publishing or transmitting material containing sexually explicit acts, etc., and Section 67-B, which deals with publishing or transmitting material depicting children sexually explicit acts or pornography in electronic form. Moreover, Sections 292 and 294 (punishment for sale, etc., of obscene material) of the Penal Code, 1860, and Sections 13, 14, and 15 of the Protection of Children from Sexual Offences Act, 2012 (POCSO) could be invoked in this regard to protect the rights of women and children.
The existing legal framework in India does not adequately address cyber offences resulting from the usage of deepfakes. It is challenging to adequately govern the use of artificial intelligence, machine learning, and deepfakes due to the absence of specific regulations on these topics in the IT Act of 2000. It could be essential to amend the IT Act of 2000 to include measures that expressly address the use of deepfakes and the penalties for their misuse to better regulate offences caused by their use. Stronger legal safeguards for people whose likenesses or photos are exploited without their permission may also be part of this, as may harsher punishments for those who produce or disseminate deepfakes with nefarious intent. The fact that deepfakes are a global problem and that effective regulation and enforcement of privacy laws will probably necessitate international cooperation and coordination are equally noteworthy. In the meanwhile, people and organizations should be cautious when confirming the legitimacy of material they come across online and mindful of the possible threats connected to deepfakes.
In the meantime, governments can do the following:
Adv. Khanak Sharma (D\1710\2023)